Why consider a career as an AIMS implementer or auditor
July 6, 2024
Table of contents
List of Services
-
IntroductionList Item 1
Key topics in this section:
The impact of Artificial Intelligence on business
Five key points on how AI is impacting businesses
-
The growing demand for AIMS professionalsList Item 2
Key topics in this section:
The rising demand for skilled AIMS lead implementers
- Key areas of transformation
- Preparing for the future of auditing
- Career opportunities and growth
- Global trends and future outlook
- Accredited certifications: A Mark of excellence for AIMS professionals
- Community and professional networks
The rising demand for skilled AIMS lead auditors
- Key areas of transformation
- Preparing for the future of auditing
- Career opportunities and growth
- Global trends and future outlook
- Accredited certifications: A Mark of excellence for AIMS professionals
- Community and professional networks
-
Career path and opportunitiesList Item 3
Key topics in this section:
Specialization opportunities in AI domains
Career path in AIMS
-
Future trends and predictions in AI management and AIMSList Item 4
Key topics in this section:
Key future trends and predictions that are expected to shape the field
-
Industry-specific regulations and compliance
Key topics in this section:
AI in healthcare
AI in finance
AI in manufacturing
AI in transportation
Emerging technologies
-
From challenges to opportunities
Key topics in this section:
Data protection
Security
Ethics
Addressing challenges with ISO/IEC 42001
Setting SMART metrics for AI systems
Continuous improvement and monitoring
Additional considerations
-
Overview of AIMS and its importance
Key topics in this section:
Defining the scope of AI systems: Enhancing cybersecurity in AIMS roles
Different types of standards
Key Functions and Elements of AIMS
Incorporating the NIST AI Risk Management Framework into the AIMS
ISO/IEC 42001: The AI management system standard
-
ISO/IEC 42001: The AI management system standard
Key topics in this section:
High-level structure of ISO/IEC 42001
Annexes
-
Ethical management of Artificial Intelligence
Key topics in this section:
Promoting ethical AI development and use
Ethics and equity
Human rights approach to AI
Privacy concerns
Bias and fairness
Accountability and transparency
Building ethical AI
-
Enhancing AI governance and innovation
Key topics in this section:
AI innovation: Driving transformation and productivity
Enabling AI governance and innovation through standards
Introduction
Artificial Intelligence (AI) simulates human intelligence processes in machines, particularly computer systems. These processes include learning (the acquisition of information and rules for using the information), reasoning (using rules to reach approximate or definite conclusions), and self-correction. In essence, AI enables machines to perform tasks that typically require human intelligence.
ISO/IEC 42001 is an international standard specifically designed to provide a comprehensive framework for the management of artificial intelligence (AI) systems. This framework aims to ensure that AI technologies are developed, deployed, and managed responsibly and ethically. By offering a structured approach to AI governance, ISO/IEC 42001 helps organizations align their AI initiatives with best practices, regulatory requirements, and ethical guidelines. Its implementation facilitates risk management, enhances transparency, and promotes trust in AI systems, making it a critical tool for organizations looking to leverage AI while maintaining compliance and integrity.
If you would like to learn more, you can read the ISO Artificial intelligence: What it is, how it works and why it matters guide

The impact of artificial intelligence on business
AI is revolutionizing industries and business operations, transforming how companies function and delivering unprecedented efficiency and innovation. Across various sectors, AI streamlines processes, enhances decision-making, and drives growth. For instance, in the scientific community, AI enables researchers to envision, predicatively design, and create novel materials and therapeutic drugs, leading to potential breakthroughs in healthcare and sustainable technologies.
AI is poised to drive impressive progress, enabling novel data analysis methods and the creation of new, anonymized, and validated data sets. This will inform data-driven decision-making and foster more equitable and efficient systems. However, while AI offers numerous advantages, it also introduces significant security challenges and societal implications that demand careful oversight and strategic management. Some experts even warn of theoretical risks associated with achieving human-level general intelligence (AGI), as these systems could potentially act in unpredictable ways.
A well-defined AI strategy is crucial for maximizing AI's impact by aligning its adoption with broader business goals. This strategy provides a roadmap for overcoming challenges, building capabilities, and ensuring responsible use. As AI continues to advance, businesses must navigate both its benefits and challenges, driving innovation while mitigating risks and addressing ethical concerns.
The increasing use of technology is fundamentally transforming business operations and audit processes. Digitization and automation drive this change, while there's a growing emphasis on sustainability, environmental, social, and governance (ESG) factors. Stakeholders like employees, investors, and customers demand comprehensive and transparent reporting on a company's performance and related risks. This shift accelerates change in the audit profession and increases the demand for skilled AIMS (Artificial Intelligence Management Systems) auditors.
Key areas of transformation
- Broadening the scope of audited data: Auditors now analyze a broader range of data beyond traditional financial information, including ESG topics, advanced technologies, and automated systems. Trustworthiness is crucial as companies report on diverse areas like climate impact, diversity and inclusion, and community engagement.
- Technology and automation in auditing: Technology and automation enable more efficient and accurate audits. AI analyzes large datasets, identifies patterns, and assesses risks, enhancing the audit process.
- Next-generation skills:
Auditors must develop next-generation skills to use new technologies and audit expanded areas effectively. Continuous learning and adaptation are essential as the profession evolves to meet the expanding needs of capital markets.
Ensuring governance and social responsibility
AIMS professionals play a vital role in fostering social responsibility and governance within organizations. They ensure AI technologies contribute positively to society and adhere to ethical standards.
- Risk management: AIMS professionals identify and mitigate risks associated with AI systems, ensuring they align with organizational governance and social responsibility objectives.
- Compliance: They ensure AI systems comply with relevant regulations, industry standards, and organizational policies, promoting governance and social responsibility.
- Promoting ethical practices: AIMS auditors champion ethical AI use, advocating for transparency, fairness, and accountability. They ensure that AI systems do not perpetuate biases or inequality, thus promoting social justice.
- Community engagement: AIMS professionals often engage with various stakeholders, including employees, customers, and the wider community. This engagement helps build trust and ensures that AI technologies meet the needs and expectations of society.
- Educational outreach:
By participating in educational initiatives, AIMS auditors help raise awareness about the ethical use of AI. They contribute to the development of guidelines and best practices that can be adopted by other professionals and organizations.
- Continuous monitoring: AIMS professionals continuously monitor AI systems to ensure they remain aligned with governance and social responsibility objectives, identifying areas for improvement.
- Collaborative networks: Professional networks allow AIMS auditors to share knowledge, stay updated on AI governance advancements, and collaborate for more effective AI management.

Rising demand for skilled AIMS lead implementers
Accredited certifications are becoming increasingly important for AIMS implementers, providing formal recognition of their skills and expertise. One such certification is the ISO 42001 Lead Implementer, designed for professionals who wish to specialize in implementing AI management systems.
Key Areas of Transformation
The ISO 42001 Lead Implementer certification equips professionals with the knowledge and skills to navigate key areas of transformation within AI management:
- AI governance frameworks:
Developing comprehensive frameworks to ensure responsible use of AI systems in compliance with regulatory standards.
- Ethical integration:
Embedding ethical principles into AI systems to prevent biases and ensure fairness and transparency.
- Data management:
Implementing robust data governance practices to protect data integrity, privacy, and security.
- Security measures: Establishing security protocols to safeguard AI systems from cyber threats and unauthorized access.
- Transparency and accountability:
Ensuring AI processes and decisions are transparent with accountability mechanisms in place.
- Continuous monitoring and improvement: Setting up systems for ongoing evaluation and enhancement of AI management practices to adapt to evolving standards and technologies.
Preparing for the Future as a Lead Implementer
To prepare for the future, AIMS implementers must focus on continuous learning and adaptability:
- Staying updated on AI trends:
Regularly update knowledge on emerging AI technologies and methodologies.
- Regulatory compliance: Keep abreast of changes in international and local regulations to ensure ongoing compliance.
- Collaborative approach: Work closely with auditors and other stakeholders to ensure well-implemented and regularly reviewed AI systems.
- Professional development: Engage in continuous education and certification programs to enhance skills and expertise.
- Innovative solutions:
Foster a culture of innovation by exploring new tools and approaches to improve AI system implementation and management.
- Risk management: Develop and implement comprehensive risk management strategies to identify, assess, and mitigate potential risks associated with AI systems.
The Role of AIMS Implementers
AIMS implementers play a crucial role in the successful deployment and governance of AI systems. They develop, establish, and maintain AI management systems that align with the ISO 42001 standard or other standards, ensuring AI technologies are used responsibly and ethically, mitigating risks, and enhancing organizational efficiency.
Career Opportunities and Growth
The demand for skilled AIMS implementers is growing across various industries, offering numerous career opportunities and potential for professional growth. Industries such as finance, healthcare, manufacturing, and technology are increasingly seeking implementers with expertise in AI management systems. Obtaining an ISO 42001 Lead Implementer certification enhances credibility and opens up new career paths.
Community and Professional Networks
Joining professional networks and communities is crucial for continuous learning and staying updated with industry best practices. Organizations such as the AI Ethics Lab , the Association for the Advancement of Artificial Intelligence (AAAI) or joining LinkedIn groups are dedicated to AI management offer valuable resources and support for AIMS Lead Implementers.
By obtaining an ISO 42001 Lead Implementer certification, professionals can play a crucial role in guiding organizations through the complexities of AI system implementation and governance. This certification shows a commitment to continuous learning and adherence to the highest standards of practice in AI management.
Rising demand for skilled AIMS lead auditors
As the audit profession evolves, its primary objective remains providing assurance over comprehensive, comparable, and objective information. AIMS auditors are crucial in this process, ensuring AI systems are used responsibly and ethically within organizations. They verify that AI technologies comply with regulations, are free from biases, and align with broader business goals.
- Independence and skepticism:
AIMS auditors maintain independence and professional skepticism, ensuring AI systems are trustworthy and the data they generate is reliable.
- Evaluating internal systems:
They assess internal systems for processing data, ensuring reported data is reliable, comparable, and relevant.
- Assuring ESG data: With the growing importance of ESG reporting, AIMS auditors ensure the accuracy of ESG data, including greenhouse gas emissions, climate-related risks, and other non-financial information.
Preparing for the future of auditing
To prepare for the future, auditing firms must invest in core auditor skills while also emphasizing new competencies required for digital transformation and ESG assurance.
- Investment in training: Companies should proactively train their professionals on ESG and emerging technologies. For example,
KPMG is investing $1.5 billion globally to train its professionals on ESG in collaboration with institutions like NYU Stern’s Center for Sustainable Business and the University of Cambridge’s Judge Business School.
- Embracing technology: Auditors must adopt technology and automation tools to enhance their capabilities, including using AI for data analysis, risk assessment, and compliance monitoring.
- Focusing on independence and integrity: Maintaining core values of independence, integrity, and professional skepticism is essential as the scope of audits expands.
Career opportunities and growth
The demand for skilled AIMS auditors is growing across various industries, offering numerous career opportunities and potential for professional growth. Industries such as finance, healthcare, manufacturing, and technology increasingly seek auditors with expertise in AI management systems. This growing demand presents a promising career path for those interested in AI governance and auditing.
Global trends and future outlook
Global trends in AI governance and auditing indicate a strong future for the profession. Emerging technologies, evolving regulatory landscapes, and the increasing importance of ESG factors are shaping the field. Staying informed about these trends and adapting to new challenges will be crucial for AIMS auditors.
Community and Professional Networks
Joining professional networks and communities is crucial for continuous learning and staying updated with industry best practices. Organizations such as the Institute of Internal Auditors (IIA), the Association for the Advancement of Artificial Intelligence (AAAI) or joining LinkedIn groups are dedicated to AI management offer valuable resources and support for AIMS auditors.
As an AIMS auditor, you will be at the forefront of a transformative era in auditing. Your role will be vital in guiding organizations through the complexities of AI management, ensuring that AI systems are innovative, productive, secure, ethical, and compliant with global standards. By fostering a culture of continuous improvement and collaboration, you will help organizations harness the full potential of AI while mitigating its risks.
Accredited certifications: A mark of excellence for AIMS professionals
Accredited certifications are gaining significance for AIMS professionals, serving as formal acknowledgment of expertise and skills. Two important certifications are:
- ISO 42001 Lead Auditor: Designed for professionals specializing in auditing AI management systems, this certification validates an auditor's ability to assess the effectiveness and compliance of AI systems against the ISO 42001 standard, ensuring they meet global benchmarks for ethical and responsible AI use.
- ISO 42001 Lead Implementer: This certification is tailored for professionals responsible for implementing AI management systems, ensuring they meet the ISO 42001 standard's requirements. It demonstrates their expertise in establishing effective AI governance, risk management, and compliance processes.
By achieving either the ISO 42001 Lead Auditor or Lead Implementer certification, professionals can:
- Enhance credibility and reputation
- Unlock new career opportunities
- Play a vital role in guiding organizations through AI governance and compliance complexities
These certifications also demonstrate a commitment to ongoing learning and adherence to the highest standards of practice in AI management, showcasing dedication to excellence in the field.
Career path and opportunities
Pursuing a career as an AIMS implementer or auditor offers numerous professional development and certification opportunities, validating your expertise and commitment to the highest standards in AI management systems. This expertise is crucial in cybersecurity, where AI systems require careful management to prevent potential vulnerabilities.
Specialization opportunities in AI domains
A career in AIMS offers various specialization opportunities across AI domains, including AI ethics, data privacy, machine learning, neural networks, and AI-driven automation. Specializing in a particular domain allows you to become an expert, opening up niche career opportunities and making you a valuable asset to any organization, particularly in cybersecurity.
Safeshield offers accredited certifications to boost your cybersecurity career
ISO/IEC 42001 Lead Implementer - Artificial Intelligence Management System
Learn MoreAs AI continues to advance rapidly, the need for effective standardization and regulation becomes crucial to ensure its responsible use. SafeShield offers the ISO/IEC 42001 Lead Implementer accredited accredited training course, designed to equip you with the skills to establish, implement, maintain, and improve an AI management system (AIMS) within an organization.
ISO/IEC 42001 provides a comprehensive framework for the ethical implementation of AI systems, emphasizing principles like fairness, transparency, accountability, and privacy. This training will prepare you to harness AI's transformative power across various industries while maintaining ethical standards.
Upon completing the course, you will have the expertise to guide organizations in leveraging AI effectively and ethically.
ISO/IEC 42001 Lead Auditor - Artificial Intelligence Management System
Learn MoreSafeShield offers an ISO/IEC 42001 Lead Auditor accredited training course designed to develop your expertise in auditing artificial intelligence management systems (AIMS). This comprehensive course equips you with the knowledge and skills to plan and conduct audits using widely recognized audit principles, procedures, and techniques.
Upon completing the course, you can take the exam to earn the "PECB Certified ISO/IEC 42001 Lead Auditor" credential, demonstrating your proficiency in auditing AI management systems.
Certified ISO/IEC 27001 Lead Implementer
Learn MoreSafeShield's ISO/IEC 27001 Lead Implementer accredited training course empowers you to develop a robust information security management system (ISMS) that effectively tackles evolving threats. This comprehensive program provides you with industry best practices and controls to safeguard your organization's information assets.
Upon completing the training, you'll be well-equipped to implement an ISMS that meets ISO/IEC 27001 standards. Passing the exam earns you the esteemed "PECB Certified ISO/IEC 27001 Lead Implementer" credential, demonstrating your expertise and commitment to information security management.
Certified ISO/IEC 27001 Lead Auditor
Learn MoreSafeShield offers an ISO/IEC 27001 Lead Auditor training course designed to develop your expertise in performing Information Security Management System (ISMS) audits. This course will equip you with the skills to plan and conduct internal and external audits in compliance with ISO 19011 and ISO/IEC 17021-1 standards.
Through practical exercises, you will master audit techniques and become proficient in managing audit programs, leading audit teams, communicating with clients, and resolving conflicts. After completing the course, you can take the exam to earn the prestigious "PECB Certified ISO/IEC 27001 Lead Auditor" credential, demonstrating your ability to audit organizations based on best practices and recognized standards.
Future trends and predictions in AI management and AIMS
As artificial intelligence (AI) continues to advance, the landscape of AI management and Artificial Intelligence Management Systems (AIMS) is poised for significant evolution. Here are some key future trends and predictions expected to shape the field:
Increased integration of AI and AIMS
Trend: The integration of AI into AIMS will become more sophisticated.
Prediction: AI-powered AIMS will automate routine monitoring and compliance tasks, allowing for real-time adjustments and predictive maintenance, increasing efficiency and reducing the burden on human managers.
Enhanced focus on ethical AI
Trend: There will be a growing emphasis on developing and deploying ethical AI systems.
Prediction: Organizations will adopt robust frameworks for ensuring fairness, accountability, and transparency in AI systems, making ethical guidelines a standard part of AIMS to mitigate biases and ensure equitable outcomes.
Strengthening of regulatory frameworks
Trend: Governments and regulatory bodies will continue to develop and refine AI regulations, such as the European Union's General Data Protection Regulation (GDPR) and the American AI Initiative.
Prediction: Compliance with AI-specific regulations will become mandatory, driving organizations to deeply integrate regulatory requirements into their AIMS, ensuring AI systems are both innovative and ethical.
Advancements in AI auditing
Trend: The auditing of AI systems will become more advanced and automated.
Prediction: AI-driven auditing tools will provide continuous monitoring and real-time reporting, enhancing the ability to detect and address issues promptly, leading to more transparent and accountable AI practices.
Focus on explainable AI
Trend: Explainability and transparency of AI systems will be prioritized.
Prediction: Explainable AI (XAI) will become a key component of AIMS, offering clear insights into AI decision-making processes, improving stakeholder trust, and facilitating compliance with regulatory standards.
Expansion of AI applications
Trend: The scope of AI applications will continue to expand across various industries.
Prediction: As AI is adopted in new domains, AIMS will need to adapt to manage industry-specific requirements and challenges, driving the development of customizable and scalable AIMS solutions.
Increased collaboration and knowledge sharing
Trend: Collaboration between organizations, academia, and regulatory bodies will intensify.
Prediction: Shared best practices, research, and case studies will help organizations improve their AI management strategies. Collaborative platforms will emerge, fostering a community approach to tackling AI challenges.
AI-Driven predictive analytics
Trend: Predictive analytics powered by AI will become a cornerstone of strategic decision-making.
Prediction: Organizations will leverage AI-driven predictive analytics to anticipate market trends, customer behavior, and operational challenges, enabling proactive management and continuous improvement of AI systems.
Emphasis on data privacy and security
Trend: Data privacy and security concerns will intensify as AI systems handle increasingly sensitive information.
Prediction: Enhanced data protection measures will be integrated into AIMS, ensuring compliance with global data privacy regulations and safeguarding against cyber threats.
Growth of AI talent and expertise
Trend: The demand for skilled AI professionals will continue to rise.
Prediction: Organizations will invest heavily in training and development programs to build AI expertise. This will include specialized roles focused on AIMS, ensuring effective management and governance of AI technologies.
By anticipating and preparing for these trends, organizations can stay ahead in the rapidly evolving field of AI management. Embracing these future directions will not only enhance the effectiveness of AIMS but also ensure the responsible and ethical deployment of AI technologies, fostering innovation and trust in AI-driven solutions.
Industry-specific regulations and compliance
AI governance must consider industry-specific regulations to ensure compliance and optimize operations. Different industries face unique challenges and regulatory landscapes that influence how AI technologies are implemented and managed.
Healthcare
Developing regulations for AI in healthcare requires a clear understanding of both AI and the unique characteristics of healthcare. The World Health Organization emphasizes the need for AI systems to prioritize patient rights over commercial interests, demanding patient-centric development. This includes considering ethical principles like autonomy and justice, and modifying regulations to allow for the use of de-identified patient data in AI-driven research.
Key considerations for AI regulations in healthcare:
- Patient-centric development: Prioritizing patient rights over commercial interests.
- Data protection and privacy: Ensuring robust measures for safeguarding patient data.
- Transparency and explainability: Making AI decision-making processes clear and understandable.
- Accountability and liability: Defining clear responsibilities and liabilities in AI applications.
- Ethical principles and fairness: Embedding ethical considerations to ensure fairness and justice in AI systems.
Ensuring compliance and safety
Regulatory agencies should develop specific guidelines, collaborate with stakeholders, and provide resources for AI vendors. Phased compliance, regular audits, and certification systems can help ensure adherence to regulations. Feedback mechanisms can refine and improve regulations over time, as demonstrated by the US FDA's regulatory framework for AI-based medical software.
Finance
Case study: How AI can be regulated in the Canadian financial sector
AI adoption in Canada's financial institutions is on the rise, with major banks and financial enterprises integrating AI technologies into consumer-facing applications.
Benefits and risks of AI in finance
AI offers significant benefits, including personalized customer experiences and better product choices. However, it also poses risks, such as:
- Lack of recourse for contesting automated decisions
- Uninformed use of AI-powered investment algorithms
To address these challenges, the Schwartz Reisman Institute for Technology and Society published a white paper recommending the leveraging of existing consumer protection laws. This approach aims to provide a framework for regulating AI in finance and mitigating potential risks.
Developing regulations for AI in finance
Currently, there are no enforceable AI regulations for the Canadian financial sector. Regulatory bodies have issued recommendations, but there is lack of enforceability. New federal legislation is being proposed to introduce comprehensive AI regulation.
Addressing AI-related risks
Consumer protection amendments to existing banking laws have introduced frameworks that address transparency, non-discrimination, oversight, and accountability. These frameworks offer a temporary solution to mitigate AI-related risks until more specific AI regulations are enacted.
Manufacturing: enhancing quality control and compliance
The manufacturing industry faces numerous challenges, including evolving industry standards and regulations. AI technologies, like machine learning and predictive analytics, can transform quality control and compliance. By analyzing production data, AI detects patterns and anomalies, ensuring product quality and compliance with standards like ISO.
Cybersecurity in AI-driven manufacturing
AI implementation in manufacturing requires a strategic approach to ensure data privacy and security. Manufacturers must:
- Protect sensitive data and comply with regulations like the EU AI Act, the GDPR, and ISO 27001
- Implement robust data systems for effective AI applications.
- Ensure secure data infrastructure and monitor AI performance.
Best practices for AI implementation
To meet evolving industry standards, manufacturers should:
- Identify priority areas for AI implementation.
- Invest in data infrastructure.
- Collaborate across functions.
- Start small and scale gradually.
- Provide training and upskilling.
- Monitor and iterate.
- Stay updated on regulatory changes.
- Embrace collaboration and partnerships.
- Cultivate a culture of innovation.
Transportation: Balancing innovation and ethics
The transportation industry has witnessed a significant transformation with the integration of AI. From autonomous vehicles to smart public transport and optimized traffic management, AI has revolutionized the way we travel. However, these advancements come with ethical concerns that need to be addressed.
Autonomous vehicles: Safety and trust
Autonomous vehicles (AVs) rely on AI algorithms to navigate roads, posing safety concerns and ethical dilemmas. Cybersecurity risks include software bugs, hacking, accident responsibility, malfunctions, and data privacy issues, which can lead to accidents and compromised passenger safety.
Decision-making algorithms in AVs face two major challenges. First, moral algorithms must be programmed to make ethical decisions in crash scenarios, prioritizing safety and resolving dilemmas such as passenger vs. pedestrian safety. Also, ensuring AI algorithms are free from biases is crucial to prevent discriminatory navigation and pedestrian recognition, guaranteeing fair and safe decision-making processes.
Moreover, public trust is essential, as AVs must prioritize safety over efficiency to ensure the well-being of passengers and pedestrians alike.
Traffic management systems: Efficiency vs. privacy
AI-driven traffic management systems offer numerous benefits, including enhanced safety and efficiency, reduced congestion, and environmental benefits. By analyzing real-time traffic patterns, these systems optimize signal timings, prevent accidents, and prioritize emergency vehicles. However, privacy and security concerns, such as surveillance, data misuse, and security risks, can erode trust and undermine public confidence. Ensuring transparency, unbiased algorithms, and robust data handling practices is crucial.
Public transport: Accessibility vs. surveillance
AI transforms public transport by improving efficiency and accessibility for all. Smart routing and scheduling, predictive maintenance, and real-time updates enhance the passenger experience. However, AI systems may inadvertently discriminate if trained on biased data.
Balancing benefits and risks
To address these concerns, the transportation industry must implement transparent data policies, stringent regulations, and ethical AI design. Engaging with the public and building trust through open communication and education is crucial. By prioritizing safety, security, and privacy alongside AI adoption, the industry can strike a balance between innovation and responsibility.
Emerging technologies
As AI converges with other technologies like blockchain, IoT, and quantum computing, new governance and innovation challenges will arise. This includes ensuring that AI systems are designed to work seamlessly with emerging technologies and that governance frameworks are adaptable to address emerging risks and opportunities.
The development and deployment of AI present a complex set of challenges, requiring a strategic approach to mitigate risks and maximize benefits.
Data Protection
Protecting data is crucial in AI, which relies on vast amounts of information, raising privacy and misuse concerns. As AI technologies advance, their ability to collect, analyze, and potentially exploit data grows, necessitating robust data protection measures. Ensuring compliance with data protection regulations and maintaining individual privacy is critical for gaining public trust and preventing misuse. Transparent data handling and data anonymization techniques are essential for safeguarding personal information.
Security
AI introduces significant security challenges. Its speed and sophistication make AI systems powerful tools but also targets for malicious use. For instance, generative AI can create deepfake videos and voice clones, spreading misinformation and disrupting societal harmony. The weaponization of AI for cyberattacks and military use poses severe risks, as does potential misuse by terrorists or authoritarian regimes. The concentration of AI development within a few companies and countries creates supply-chain vulnerabilities. Developing AI systems with built-in security features and continuous monitoring for vulnerabilities is essential to mitigate these risks.
Ethics
Ethical considerations are critical in AI development and deployment. AI systems can reinforce biases present in their training data, leading to discriminatory outcomes in hiring and law enforcement. As AI becomes more integrated into decision-making, ensuring fairness, transparency, and accountability becomes increasingly important. The potential for AI to achieve human-level general intelligence (AGI) amplifies ethical concerns, as such systems could act unpredictably and harm society. Developing ethical guidelines and frameworks for AI use and ensuring compliance through audits and assessments are essential to mitigate ethical risks.
Setting SMART metrics for AI systems
To maximize the potential of AI systems, it's crucial to establish clear and measurable objectives. Setting SMART metrics provides a framework for tracking progress and achieving tangible improvements.
To track and measure the success of AI systems, SMART metrics should be applied:
- Specific: Clearly define goals that focus on precise aspects or outcomes of the AI system, avoiding ambiguity.
- Measurable: Set quantifiable objectives with concrete metrics or key performance indicators (KPIs) to track progress and evaluate success.
- Achievable: Ensure goals are realistic and attainable within the organization’s resources, capabilities, and constraints, considering technology readiness, expertise, and budget.
- Relevant: Align objectives with the organization’s overall goals, strategic priorities, and mission to ensure they address key business challenges or opportunities.
- Time-bound:
Establish a defined timeframe for achieving objectives, creating a sense of urgency and enabling effective progress monitoring.
Aligning objectives with stakeholder expectations
After applying SMART metrics, it is crucial to consider the expectations and needs of various stakeholders, including customers, employees, investors, and regulatory bodies.
By setting SMART metrics, organizations can:
- Track progress and measure success
- Focus efforts on achieving tangible improvements
- Align AI objectives with business strategy
- Optimize resource allocation and ROI
Example SMART metrics for AI systems include:
- Reduce process time by 30% through automation within the next year
- Achieve a 20% increase in sales leads generated through AI-driven marketing efforts within the next quarter
Continuous improvement and monitoring
A robust cycle of continuous improvement is essential for addressing AI challenges and ensuring ongoing compliance. This approach involves regularly reviewing and enhancing AI systems and processes to adapt to evolving risks and regulatory requirements. Continuous monitoring helps identify and mitigate biases, security vulnerabilities, and ethical concerns promptly. Iterative improvements maintain data protection standards and align AI systems with the latest privacy regulations. A culture of continuous improvement fosters innovation while reinforcing accountability and transparency in AI operations. Regular updates and audits enable organizations to stay ahead of emerging threats and maintain compliance with standards.
Additional Considerations
- Investment in AI research: Funding research initiatives supports the development of new AI technologies and applications that benefit society.
- Workforce re-skilling: Investing in education and training programs prepares workers for new roles created by AI technologies.
- Public awareness and education: Raising awareness about AI risks and ethical considerations fosters responsible use and builds public trust.
- Human oversight and governance: Effective governance structures and human oversight ensure responsible AI use, maintaining accountability and ethical standards.
- Collaboration and standardization: International collaboration and standardization help address global AI challenges, ensuring consistency and coordination across borders. Establishing common standards and guidelines for AI use promotes safe, ethical, and effective AI technologies.
Artificial Intelligence Management Systems (AIMS) offer a structured approach to managing and optimizing AI systems within organizations. These systems emphasize ethical considerations, such as fairness and accountability, and provide centralized tools to enhance AI governance.
As AI capabilities grow, concerns about privacy, bias, inequality, safety, and security become more pressing. AIMS address these issues by guiding organizations on their AI journey, ensuring responsible and sustainable deployment of AI technologies.
Defining the scope of AI systems: Enhancing cybersecurity in AIMS roles
As AI technologies continue to evolve, defining a clear scope for their implementation within organizations is crucial. This ensures alignment with strategic goals, business processes, and most importantly, cybersecurity protocols. AI systems encompass various technologies, including chatbots, predictive analytics, and fraud detection, each with unique requirements, risks, and potential vulnerabilities.
Cybersecurity risks associated with AI systems include data poisoning, model inversion attacks, and unauthorized access. It is essential to involve stakeholders from cybersecurity, data science, and business operations in defining and managing AI systems. Regular reviews and updates ensure AI systems remain aligned with organizational goals and cybersecurity protocols.
A well-defined scope provides a roadmap for implementation, operation, and monitoring, helping identify necessary resources, potential risks, and mitigation strategies. By defining the scope of AI systems, organizations can better prepare for AI adoption's challenges and opportunities, ultimately strengthening their cybersecurity resilience.
Different types of standards
The rapid development of AI standards has led to a comprehensive framework covering various applications relevant to AI governance and innovation. The AI Standards Hub Database includes numerous standards that codify technical specifications, measurement, design, and performance metrics for products and systems. These standards ensure that AI technologies are safe, effective, and compliant with regulatory requirements, fostering trust and enabling widespread adoption.
Key Functions and Elements of AIMS
Risk and opportunity management
- Identify and manage risks: Identify and manage AI-related risks and opportunities.
- Trustworthiness of AI systems: Ensure AI systems are secure, safe, fair, transparent, and maintain high data quality throughout their lifecycle.
Impact assessment
- Impact assessment process: Assess potential consequences for users of the AI system, considering technical and societal contexts.
- System lifecycle management: Manage all aspects of AI system development, including planning, testing, and remediation.
AI governance and performance optimization
- Define and facilitate AI governance: Establish clear objectives and policies for AI governance.
- Optimize deployment and maintenance: Enhance the deployment and maintenance of AI models.
- Foster collaboration: Promote teamwork between different teams.
- Provide dynamic AI reports: Generate dynamic reports for better oversight and decision-making.
- Performance optimization: Continuously improve the effectiveness of AI management systems.
Data quality and security management
- Ensure regulatory compliance: Adhere to relevant regulations and standards.
- Guarantee accountability and transparency: Maintain transparency and accountability in AI operations.
- Identify and mitigate risks: Recognize and address AI-related risks.
Supplier management
- Oversee suppliers and partners: Manage relationships with suppliers, partners, and third parties involved in AI system development and deployment.
Continuous improvement and monitoring
- Continuous improvement: Implement processes for ongoing improvement of AI systems.
- Performance monitoring: Continuously monitor AI system performance and impact.
Ethical considerations
- Ethics and fairness: Integrate ethical principles and ensure fairness in AI operations.
- Ethical AI design: Ensure inclusive and ethical AI design, overseen by ethical review boards.
User training and support
- Training programs: Develop and deliver training programs for users and stakeholders.
- Support systems: Provide ongoing support and resources for effective AI system utilization.
Compliance and legal monitoring
Stay updated on legal changes: Regularly monitor changes in laws and regulations related to AI.
Legal risk management: Assess and manage legal risks associated with AI deployment.
Stakeholder engagement
- Engage with stakeholders: Communicate with stakeholders to gather feedback and ensure alignment.
- Public reporting: Transparently report AI activities to stakeholders and the public.
Sustainability and environmental impact
- Assess environmental impact: Evaluate and minimize the environmental impact of AI systems.
- Sustainable practices: Implement sustainable practices in AI development and deployment.
User experience and human-centered design
- User-centered AI design: Design AI systems that prioritize user experience.
- Feedback mechanisms: Implement feedback mechanisms to improve AI systems based on user input.
Incorporating the NIST AI Risk Management Framework into the AIMS
The National Institute of Standards and Technology (NIST) is part of the U.S. Department of Commerce. NIST’s mission is to promote U.S. innovation and industrial competitiveness by advancing measurement science, standards, and technology to enhance economic security and improve quality of life.
Directed by the National Artificial Intelligence Initiative Act of 2020, the NIST AI risk management framework (AI RMF) aims to assist organizations in managing AI risks and promoting trustworthy AI development and use. This voluntary, rights-preserving framework is non-sector-specific and adaptable for organizations of all sizes and sectors.
Foundational information: The first part of the NIST AI RMF outlines essential concepts for understanding and managing AI risks, such as risk measurement, tolerance, and prioritization. It also defines characteristics of trustworthy AI systems, emphasizing validity and reliability across contexts, safety for human life and the environment, resilience to attacks, transparency and accountability, clear decision-making explanations, user privacy protection, and fairness to avoid bias.
AI RMF core: The AI RMF core includes four primary domains to help AI actors manage AI risks effectively:
- Govern: Build a risk management culture within organizations through processes, documentation, and organizational schemes.
- Map: Establish context for AI systems by understanding their purposes, impacts, and assumptions, and engage stakeholders for risk identification.
- Measure: Provide tools and practices for analyzing and monitoring AI risks using quantitative and qualitative methods.
- Manage: Implement strategies for AI risk treatment and mitigation.
AI RMF profiles: AI RMF profiles are tailored implementations of the AI RMF core functions for specific contexts, use cases, or sectors. Types include:
- Use-case profiles: Custom implementations for particular use cases, such as hiring or fair housing.
- Temporal profiles: Describe the current and target states of AI risk management within a sector.
- Cross-sectoral profiles: Address risks common across various sectors or use cases, such as large language models or cloud-based services.
The NIST AI 100–1 offers a flexible framework for understanding and managing AI risks. This framework, divided into foundational information and core domains—Govern, Map, Measure, and Manage—enhances accountability and transparency in AI system development when integrated into organizational practices.
ISO/IEC 42001 follows a high-level structure with 10 clauses:
- Scope:
Defines the standard's purpose, audience, and applicability.
- Normative references:
Outlines externally referenced documents considered part of the requirements, including ISO/IEC 22989:2022 for AI concepts and terminology.
- Terms and definitions:
Provides key terms and definitions essential for interpreting and implementing the standard.
- Context of the organization: Requires organizations to understand internal and external factors influencing their AIMS, including roles and contextual elements affecting operations.
- Leadership: Requires top management to demonstrate commitment, integrate AI requirements, and foster a culture of responsible AI use.
- Planning: Requires organizations to address risks and opportunities, set AI objectives, and plan changes.
- Support:
Ensures necessary resources, competence, awareness, communication, and documentation for establishing, implementing, maintaining, and improving the AIMS.
- Operation:
Provides requirements for operational planning, implementation, and control processes, including AI system impact assessments and change management.
- Performance Evaluation:
Requires monitoring, measuring, analyzing, and evaluating the AIMS performance, including conducting internal audits and management reviews.
- Improvement: Requires continual improvement of the AIMS through corrective actions, effectiveness evaluations, and maintaining documented information.
The standard includes 38 controls and 10 control objectives, which organizations must implement to comprehensively address AI-related risks, from risk assessment to the implementation of necessary controls.
Annexes:
Annex A: Reference control objectives and controls
Provides a structured set of controls to help organizations achieve objectives and manage AI-related risks. Organizations can tailor these controls to their specific needs.
Annex B: Implementation guidance for AI controls
Offers detailed guidance on implementing AI controls, supporting comprehensive AI risk management. Organizations can adapt this guidance to fit their unique contexts.
Annex C: Potential AI-related organizational objectives and risk sources
Lists potential organizational objectives and risk sources pertinent to AI risk management. Organizations can select relevant objectives and risk sources tailored to their specific context.
Annex D: Use of the AI Management system across domains or sectors
Explains the applicability of the AI management system in various sectors, such as healthcare, finance, and transportation. Emphasizes the need for integration with other management system standards to ensure comprehensive risk management and adherence to industry best practices.
AI ethics refers to the principles guiding the development and use of AI systems to ensure they are fair, transparent, accountable, and beneficial for society.
Promoting ethical AI development and use
In no other field is the ethical compass more crucial than in artificial intelligence (AI). The way we work, interact, and live is being reshaped at an unprecedented pace. While AI offers significant benefits across many areas, without ethical boundaries, it risks perpetuating biases, fueling divisions, and threatening fundamental human rights and freedoms.
Ethics and equity
AI systems can impact users differently, with some populations being more vulnerable to harm. Biases in AI algorithms, especially in large language models (LLMs), can perpetuate inequities if not addressed. These models learn from their training data, which means any biases in the data can be reflected in the AI's outputs. This can lead to inaccurate, misleading, or unethical information, necessitating critical evaluation to avoid reinforcing discrimination and inequities.
Human rights approach to AI
According to UNESCO, there are ten core principles that form the basis of an ethics of AI approach based on human rights:
- Proportionality and do no harm: AI should not exceed what is necessary to achieve legitimate aims, and risk assessments should prevent potential harms.
- Safety and security:
AI systems should avoid unwanted harms and vulnerabilities to attacks.
- Right to privacy and data protection:
Privacy must be protected throughout the AI lifecycle, with robust data protection frameworks in place.
- Multi-stakeholder and adaptive governance:
Inclusive governance involving diverse stakeholders ensures that AI development respects international laws and national sovereignty.
- Responsibility and accountability:
AI systems should be auditable and traceable, with oversight mechanisms to ensure compliance with human rights norms.
- Transparency and explainability: AI systems must be transparent and their decisions explainable, balancing this with other principles like privacy and security.
- Human oversight and determination: Ultimate responsibility for AI decisions should remain with humans.
- Sustainability:
AI technologies should be assessed for their sustainability impacts, including environmental effects.
- Awareness and literacy:
Public understanding of AI should be promoted through education and engagement.
- Fairness and non-discrimination: AI should promote social justice and be accessible to all, avoiding unfair biases.
Privacy concerns
AI systems often rely on large datasets, raising significant privacy concerns. Ethical AI development must prioritize data protection and consent, ensuring individuals' privacy rights are respected and safeguarded. Transparent data handling practices and robust anonymization techniques are crucial for protecting personal information.
Bias and fairness
AI systems can inherit biases from their training data, leading to discriminatory outcomes. In areas like hiring and law enforcement, ensuring fairness and equity in AI algorithms is essential. Developers must actively work to identify and mitigate biases, striving to create AI systems that promote inclusivity and fairness.
Accountability and transparency
As AI systems take on more decision-making roles, accountability, and transparency become critical. Clear frameworks must be established to ensure that AI decision-making processes are transparent and that accountability for AI-driven decisions is maintained. This helps build public trust and ensures individuals can seek redress when affected by AI outcomes.
Building ethical AI
Promoting ethical AI development and use involves addressing several key areas:
- Transparency and oversight: Ensuring AI tools are developed with safeguards to protect against inaccuracies and harmful interactions.
- Political and social impact:
Protecting against the use of AI to spread misinformation or discriminatory content.
- Environmental impact: Assessing and mitigating the energy consumption and environmental effects of AI systems.
- Diversity and fairness:
Ensuring AI tools avoid bias and are accessible to all. Promoting inclusivity and fairness in AI development helps prevent discrimination and ensures equitable benefits.
- Privacy and data governance:
Establishing clear guidelines on how user data is used, stored, and shared, while ensuring technical robustness and safety.
- Regulatory compliance:
Adhering to local and international regulations and standards is essential for ethical AI development.
- Collaboration and partnerships:
Collaboration between governments, academia, industry, and civil society is crucial for promoting ethical AI.
- Balancing innovation and ethics:
Balancing innovation with ethical considerations is key to advancing AI technology responsibly.
- Education and training: Ongoing education and training for AI developers, users, and policymakers are vital for understanding and addressing ethical challenges.
Enhancing AI governance and innovation
AI Innovation: Driving transformation and productivity
Artificial intelligence (AI) is a transformative force, offering unprecedented opportunities for innovation and productivity enhancements. As AI continues to evolve, it reshapes the way we work, interact, and live, much like the transformative impact of the printing press centuries ago.
- Impact on employment: AI is predicted to affect up to 80% of jobs, signaling significant shifts in workforce dynamics and demanding new skills and roles.
- Productivity enhancement:
Organizations can expect up to a 30% improvement in productivity through the adoption of AI technologies. AI enables the automation of routine tasks, freeing up human workers for more complex and creative activities.
- Model versatility:
Platforms like AWS allow the use of multiple AI models within the same use case, providing flexibility and optimization opportunities. Customers can seamlessly switch between AI models to adapt to evolving requirements and performance benchmarks.
- Security measures: Robust security mechanisms, such as those offered by Bedrock, ensure the integrity and confidentiality of AI models, balancing innovation with risk mitigation.
Enabling AI governance and innovation through standards
Standards play a critical role in AI governance and innovation, providing common rules and guidelines that ensure AI systems are safe, ethical, and legally compliant. Developed through consensus in recognized Standards Development Organizations (SDOs) such as ISO, IEC, IEEE, and ITU, these standards support organizations in managing risks and building public trust.
- Global governance and market access: Standards help organizations demonstrate compliance with best practices and regulatory requirements, facilitating easier access to global markets. They ensure products meet expectations of safety and interoperability, fostering global regulatory interoperability.
- Risk management and public trust:
By providing voluntary good practice guidance and underpinning assurance mechanisms like conformity assessments, standards help manage risks and build public trust. Labels like the European CE mark demonstrate conformity with relevant standards and regulations.
- Accountability and liability:
As AI systems make decisions that impact individuals and society, there needs to be clarity on accountability and liability. This includes establishing legal frameworks that define responsibility and accountability for AI decisions, ensuring that there are mechanisms in place for redress and remediation.
- Global cooperation:
AI governance is a global issue, and international cooperation is essential to ensure consistency and coordination. This includes collaboration on standards' development, sharing best practices, and establishing common guidelines for AI development and deployment.
- Efficiency and innovation: Standards reduce costs and time involved in achieving regulatory compliance and market access, enabling organizations to innovate more efficiently. They provide clear, repeatable guidance, minimizing errors and increasing productivity.
Conclusion
The landscape of AI management and Artificial Intelligence Management Systems (AIMS) is quickly evolving, driven by technological advancements and increasing regulatory demands. Organizations need to adopt a structured approach to AI governance through standards like ISO/IEC 42001. These frameworks not only ensure ethical and responsible AI deployment but also enhance operational efficiency, data security, and compliance with global standards.
As AI continues to transform industries, the role of AIMS implementers and auditors becomes increasingly vital. Artificial Intelligence Management Systems professionals are at the forefront of ensuring that AI systems are trustworthy, transparent, and aligned with strategic goals. Their expertise helps organizations navigate the complexities of AI governance, mitigating risks and maximizing benefits.
Future trends in AI management indicate a growing emphasis on ethical AI, enhanced regulatory frameworks, and the integration of AI with other emerging technologies. By anticipating these trends and fostering a culture of innovation and ethical responsibility, organizations can harness the full potential of AI while safeguarding against its risks.
In conclusion, pursuing a career as an AIMS implementer or auditor not only offers a promising path for professional growth, but also positions individuals as key players in the responsible advancement of AI. Embracing the principles of ethical AI management, staying abreast of industry trends, and obtaining relevant certifications will empower professionals to make significant contributions to their organizations and society at large. As we move forward, the collective effort of skilled AIMS professionals will be instrumental in shaping a future where AI technologies are used to their fullest potential, with integrity and accountability at the core.
Share this article
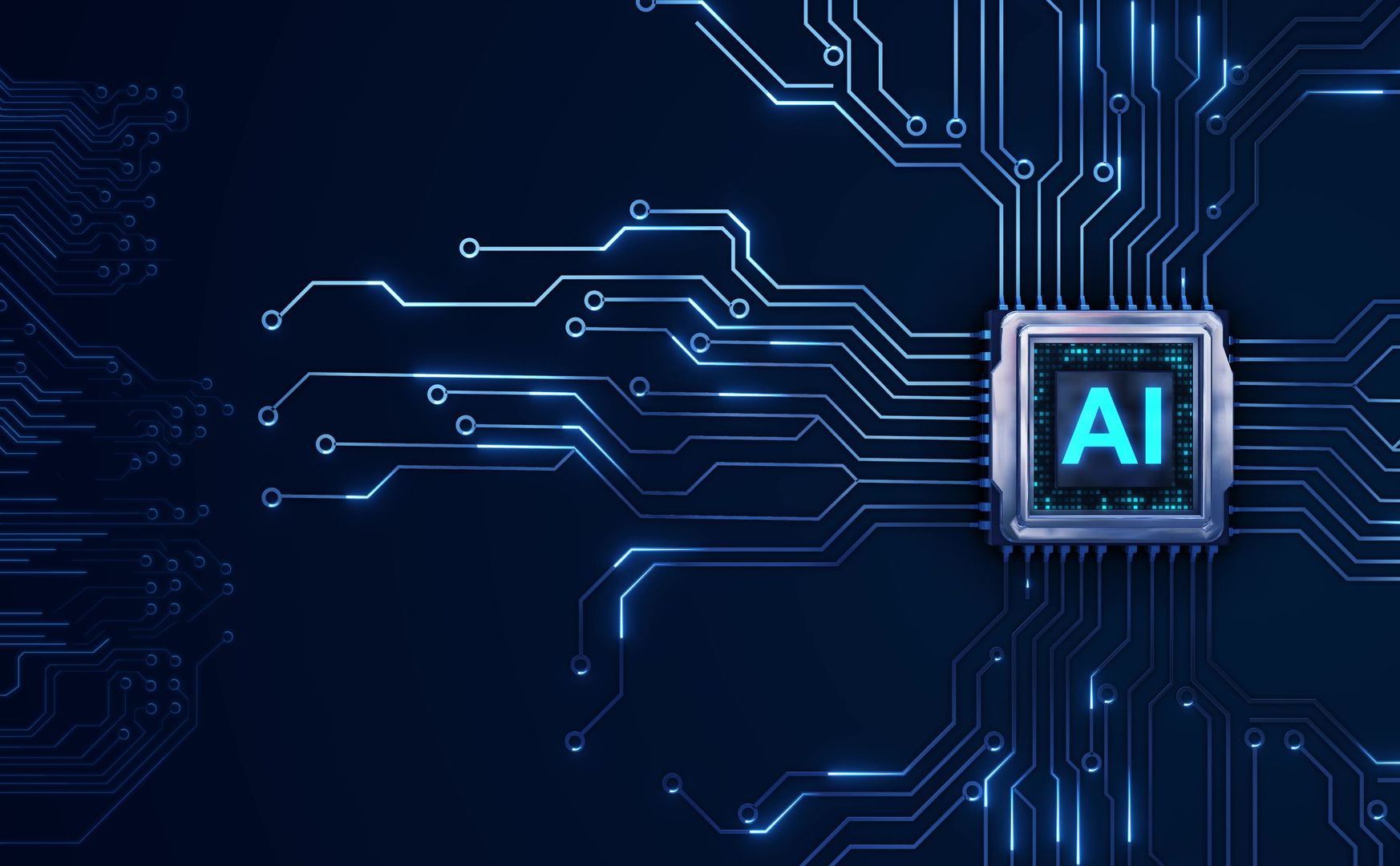